2023 is all about ChatGPT. This AI solution has gained unprecedented popularity amassing a million users in a mere five days!
The recent introduction of ChatGPT's Enterprise version holds the promise of transforming business operations and boosting profitability. However, as fine-tuning techniques evolve, it's high time for forward-thinking business leaders to carefully consider the pros and cons before embarking on their journey into generative AI (gen AI).
In this article, we will delve into the intricacies of both options, then analyze the core differences and features to guide you in choosing which solution works best for your business.
Understanding the Basics
1. What is ChatGPT?
Created by OpenAI, ChatGPT is an advanced language model trained on a massive dataset of over one trillion points (GPT-4). It learned from various sources like books, articles, and websites to understand how humans use language and generate coherent, relevant responses to different prompts.
With its ability to understand and generate human-like text, ChatGPT became a valuable tool for businesses across various industries; from customer support, sales, and marketing to financial analysis, risk assessment, and fraud detection.
2. Introducing ChatGPT Enterprise
As the name suggests, ChatGPT Enterprise is specifically designed for larger organizations that require a more customizable and controlled Gen AI solution. It was officially launched on 28 August 2023, offering the next-level AI solution tailored to enterprises.
The Enterprise version promises fast and unlimited access to GPT-4, enhanced data processing capabilities, and diverse customization features. The customizability feature will enable businesses to fine-tune the model according to their specific industries, ensuring more accurate and relevant outputs.
The major highlight of ChatGPT Enterprise was addressing the privacy and security concerns companies had when it was first rolled out in late 2022. Corporations like Samsung banned their employees from accessing ChatGPT after some of them used it to develop a company code which resulted in a leak of sensitive information.
OpenAI reported that the Enterprise version is designed with advanced security and privacy measures that guarantee customer prompts and company data full ownership, data encryption, and SOC 2 compliance.
3. What is Fine-tuned ChatGPT?
The fine-tuned ChatGPT is a pre-trained version of ChatGPT models (GPT4 or GPT-3.5 turbo) that has been further customized and optimized for specific use cases or domains. This option works well for businesses that require a more focused and tailored Gen AI experience.
Unlike ChatGPT Enterprise or ChatGPT Plus (the $20 monthly subscription), which are packaged solutions, the fine-tuned ChatGPT is a hands-on approach. It is a process by which data scientists and developers can access GPT models, through API calls, and use custom data to train them.
Why would ChatGPT need fine-tuning? Think of ChatGPT as a General Practitioner (GP) in medicine: knowledgeable but general. Sometimes, you need a specialist, like a cardiologist. Just as a GP isn't a heart specialist, ChatGPT needs a deeper dive into niche subjects.
ChatGPT models were trained on data that is not particularly relevant to your business. Any response to your specific prompts will be generic. That's where fine-tuning comes in. It's like giving our GP some specialized training, ensuring that when expertise is needed, it delivers spot-on.
When you train the model on domain-specific data, the fine-tuned ChatGPT will provide you with more accurate and context-aware responses. For example, a healthcare organization can fine-tune ChatGPT to understand medical terminology, enabling it to provide accurate and reliable information to patients.
Understanding the Key Differences
While both ChatGPT Enterprise and fine-tuned ChatGPT have their advantages, it is essential to recognize the nuances between the two options to make an informed decision. The information publicly available on ChatGPT Enterprise is still limited. However, these are the main factors to consider based on what we know so far:
1. Data Privacy, Security, and Compliance
ChatGPT Enterprise comes with built-in security, confidentiality, and compliance features. OpenAI guarantees that your data will remain encrypted and won't be used to refine its models. This is a big win for businesses that place a premium on data privacy.
On the other hand, opting for Fine-tuned ChatGPT means working with OpenAI's non-enterprise models. This implies there are no guarantees that the data you provide for fine-tuning won't contribute to OpenAI's future model training.
As always, gauge the data's sensitivity before embarking on the fine-tuning journey and determine if the security provisions align with your business needs.
2. Customization & Flexibility
ChatGPT Enterprise offers a high degree of customizability. It provides unlimited access to GPT-4, 32k token context windows, and free credits to use their APIs for further customization. This means that companies are able to “hyper-customize” OpenAI’s most advanced model based on their own data and their specific requirements, with no limits.
Similarly, fine-tuned ChatGPT is pre-trained with added customization, focusing on specific domains to understand industry-specific jargon and handle domain-specific queries. However, OpenAI API comes with 8k token context windows (for GPT-4), rate limits, and costs per 1k tokens.

3. Scalability and Integration
Integration capabilities are crucial to ensure a seamless implementation of the chosen ChatGPT option into your existing systems and workflows.
For businesses that require seamless integration with their existing systems and have a high number of user interactions, ChatGPT Enterprise comes with unique features for large-scale deployments such as an admin console with bulk member management, SSO, domain verification, and an analytics dashboard for usage insights. The model is designed to be scalable, allowing large organizations to efficiently manage and scale their conversational AI systems as their customer base grows.
On the other hand, fine-tuned ChatGPT offers flexibility in deployment but might necessitate extra resources for scaling based on your needs. While it can still handle a considerable amount of traffic, businesses with rapidly growing customer bases will need to allocate more resources to ensure optimal performance and responsiveness.
It is important to evaluate your business's scalability needs and consider the potential growth trajectory when evaluating the two options.
4. Performance and Speed
In Large Language Models (LLMs) like ChatGPT, there's a balance to strike between speed and reliability. Large-size models tend to be slower in response, but they are often more reliable. This forces a trade-off between the reliability needed from the model and the speed of the response.
In general, the response time for each request depends on many factors: internet speed, the GPT model selected, and how busy OpenAI's system is at that moment.
With fine-tuned ChatGPT, the speed and performance differ based on which GPT you choose as a base model. GPT-4 stands out in terms of accuracy, but because it's a larger model, it takes a little longer to respond. Do note however that the actual latencies are not indicated by OpenAI.
On the flip side, ChatGPT Enterprise promises a high-speed performance for GPT-4 (up to 2x faster). Tests to verify this claim haven't been conducted yet, so we'll take their word for it for now.
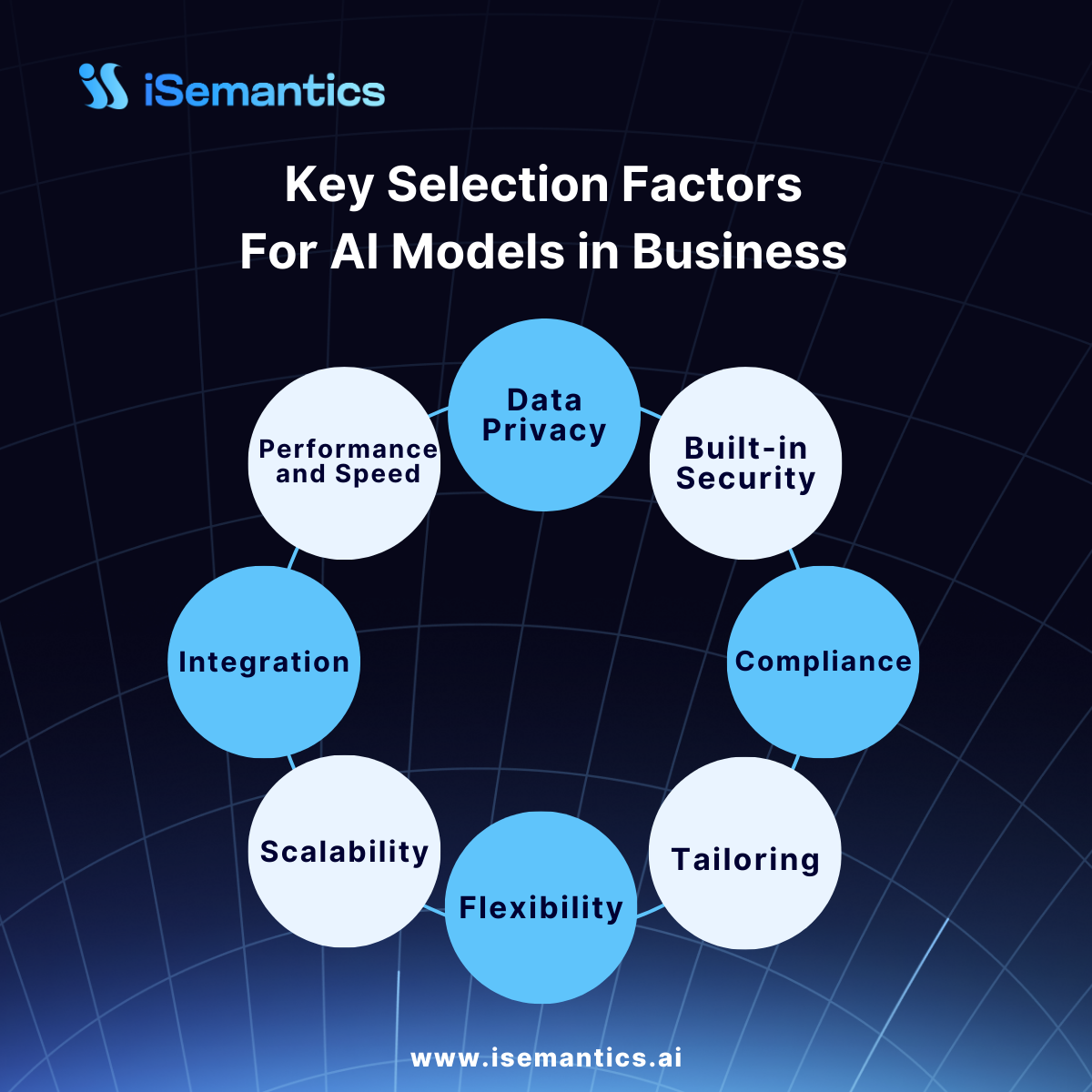
Cost Implications and Risks Associated
While the benefits of ChatGPT Enterprise and fine-tuned ChatGPT are significant, it is crucial to consider the cost implications and potential risks involved in implementing these options
1. Cost Considerations
ChatGPT Enterprise, being a more customizable and large-scale solution, comes more likely at a much higher cost compared to ChatGPT Plus or fine-tuned ChatGPT.
OpenAI has been discreet about its Enterprise pricing. However, a user on Reddit shared a quote of US$60 per user per month, for a commitment of at least 150 seats and a year-long contract.

On the other hand, a fine-tuning process is mainly used to reduce the costs of using an LLM. It allows businesses to shorten their prompts while maintaining the same performance.
With fine-tuned ChatGPT, the total cost depends mainly on the GPT model selected (cost per 1k token) and the specific use case (task complexity). In general, the fine-tuning process involves four types of costs: inference cost, tuning cost, pre-training cost, and hosting cost.

If we use the Reddit user’s pricing as a benchmark, ChatGPT Enterprise emerges as a premium offering, possibly reserved for top-tier corporations. As always, assess the potential return on investment (ROI) and long-term financial advantages before making your final choice.
2. Risk Mitigation
The risks associated with ChatGPT revolve around its limitations, be it Enterprise or the fine-tuned version since both use the same GPT-4 as the base model (although older versions can be used for fine-tuning). Both may occasionally provide incorrect or unintended responses (hallucinations), which could impact your operations, customer satisfaction, and brand reputation if not managed effectively.
According to OpenAI, “despite its capabilities, GPT-4 has similar limitations as earlier GPT models. Most importantly, it still is not fully reliable (it “hallucinates” facts and makes reasoning errors).”
Mitigating these risks involves putting protocols in place to prevent any incidents and keep the deployed ChatGPT model always in check.
- Grounding Additional Context: The model can be enhanced by connecting it to external data sources. This addition will provide added context to the responses it generates and not only limited to the data on which it was trained.
- Proactive Monitoring: It is vital to monitor system interactions with ChatGPT to pinpoint and rectify hallucinations and inaccuracies. By staying vigilant, you will be able to quickly address issues and maintain high accuracy constantly.
- Continuous Improvement: Keeping ChatGPT at its best involves a commitment to regular updates and fine-tuning. You need to keep feeding the model new data and utilize user feedback as a compass to refine the model, reduce hallucinations, and enhance its performance.
- Human Oversight: Despite ChatGPT's robust capabilities, you need to establish clear guidelines for human review and intervention to prevent any potential pitfalls.
- Avoid High-Stakes Uses: Exercise prudence especially in high-stakes contexts.
It's worth noting that with GPT-4, the frequency of inaccuracies has dropped considerably when compared to the old version. It outperforms the previous GPT-3.5 model by 40%, which itself has been improving with each iteration.
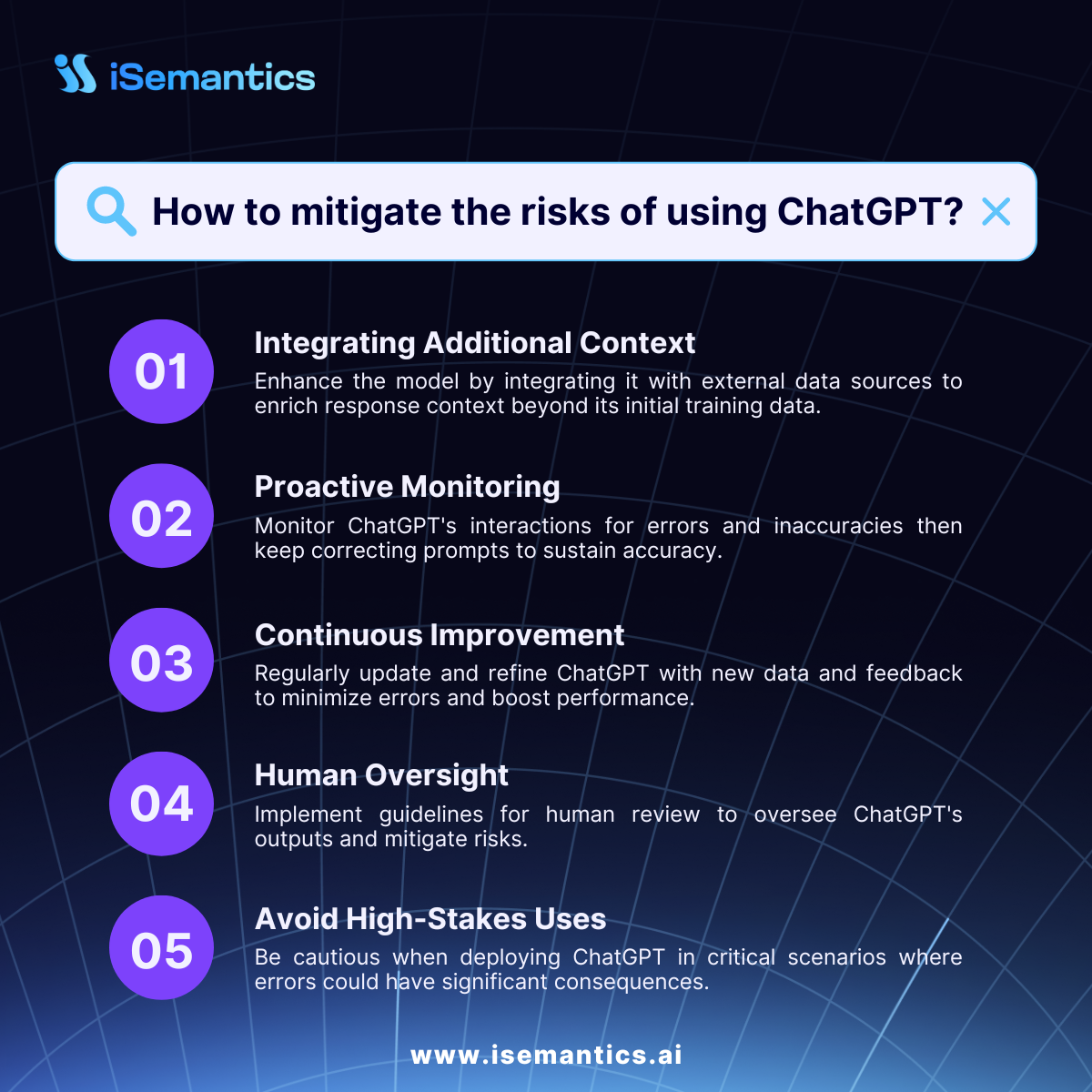
Final Thoughts
When choosing between ChatGPT Enterprise and fine-tuned ChatGPT, there is no single answer. Businesses often grapple with balancing costs and data privacy concerns. While OpenAI has hinted at introducing a self-serve ChatGPT Business for smaller teams, it remains unclear whether it will uphold Enterprise-grade data privacy and security.
The silver lining, however, is the existence of alternative solutions. Exploring fine-tuning for LLMs beyond ChatGPT opens avenues to achieve both data privacy and cost-effectiveness.
Although ChatGPT Enterprise has so far, the highest performance in terms of reliability and accuracy, limitations persist in handling multiple tasks while also excelling at individual ones. This is where the strength of smaller language models like BERT shines, particularly in individual tasks such as sentiment analysis, named entity recognition, or fake news detection, thanks to its robust natural language understanding capabilities.
In the end, the ultimate goal is to ensure the best outcomes, regardless of which LLM or which version you use.
If you're seeking further guidance on Gen AI solutions tailored to your company, our team of AI specialists is ready for consultation. At iSemantics, our commitment is to ensure that every business, regardless of size or budget, has access to the transformative power of AI.