In the competitive realm of business, every enterprise is always on the lookout for that edge, that secret sauce that can set them apart. It's the quest for enhanced competitiveness, greater profitability, and operational optimization that fuels the demand for the application of Large Language Models (LLMs), like ChatGPT, in business settings.
LLMs, with their ability to generate human-like text, are revolutionizing communication and problem-solving for businesses. ChatGPT's virality is a perfect example with over 1.4 billion monthly visits and more than 180 million users worldwide!
However, to fully unlock their potential and derive maximum value, it's essential to explore how LLMs, namely ChatGPT, can provide real value to your business.
The Fundamentals of ChatGPT and LLMs
LLMs, like ChatGPT, are essentially the superstars of AI models. They've been through a rigorous training process involving vast quantities of text data from the internet.
LLMs come pre-trained with knowledge on a wide range of topics, but their responses are usually generic. They're like a buffet table with a bit of everything but not exactly your favorite dish. To make them adapted to your business context, you'll need to serve them a customized platter – your own business data.
Before we dive deeper, let’s understand the different training techniques used in LLMs.
1. Training, Fine-tuning, RAG or Prompt Engineering
Training
Training your own foundation model means re-training an LLM from the ground up on new data to make it domain-specific. While this results in accurate output, you need an extensive repository of high-quality data, teams of AI experts, and significant computational resources. This makes it a less feasible option for many companies, not least small and medium businesses (SMBs).
Fine-tuning
Fine-tuning an existing LLM, on the other hand, is like making alterations to an off-the-rack suit to fit you better. It involves taking a pre-trained LLM and incorporating specific domain-related content (your business data) into a model already well-versed in general knowledge and language-based interaction.
This fine-tuning process involves tweaking certain parameters of the base model and typically relies on significantly less data, often only a few hundred or thousand documents, as opposed to the millions or billions necessary to build a new model from scratch. While it's less data-hungry and resource-intensive than full training, it still calls for some AI expertise.
Retrieval Augmented Generation (RAG)
For RAG, think of it as your LLM's personal librarian. It combines a pre-trained LLM with an external retrieval system that holds your company's private data. When you ask a question, the LLM fetches the most relevant information from your knowledge library to generate the response.
Prompt Engineering
This technique involves enhancing the original model through contextual prompts enriched with domain-specific knowledge to get efficient responses to related queries. However, it requires iterative efforts and careful wording due to its sensitivity to prompt phrasing.
Choosing the right technique is not a one-size-fits-all decision. It depends on various factors, including accuracy, implementation complexity, total cost, flexibility, and operational effort required by the specific technique.
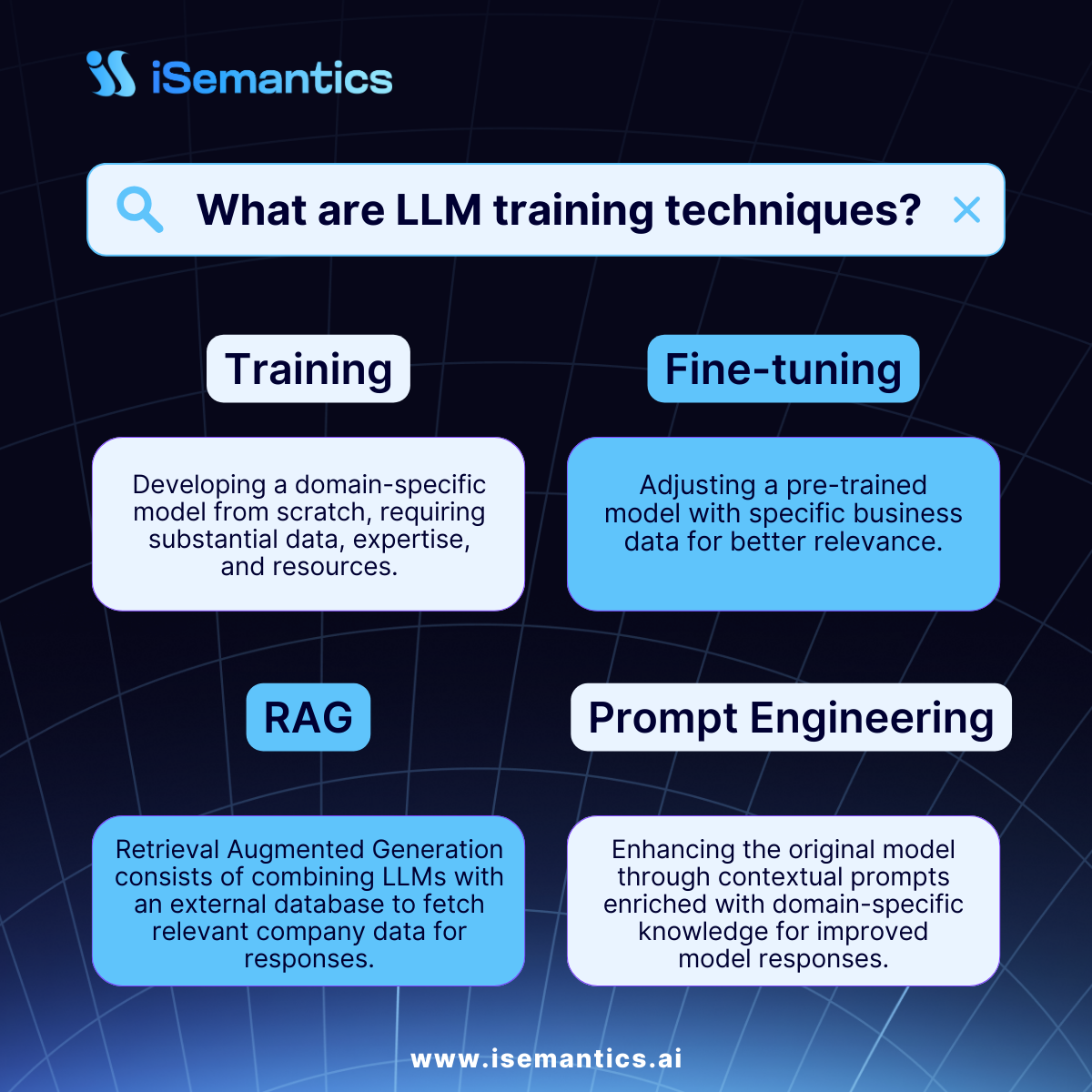
2. Why Training Matters
Training your ChatGPT or LLMs on your business data isn't just a nice-to-have; it's a game-changer. It's like giving them an insider pass to your specific industry, products, and services. This allows LLMs to generate responses that are not only coherent but also highly relevant to your unique needs.
What's even more remarkable is that training allows you to tackle the unique challenges and requirements of your business. Be it compliance regulations, product specifics, or troubleshooting protocols, these models can be fine-tuned to handle them with precision. This level of customization ensures that AI-powered conversations align perfectly with your business goals and cater to your customers' needs.
Training LLMs on your company’s data is an iterative process. As your business evolves and new data becomes available, you should keep updating and refining the models. This way, you stay ahead of the competition and deliver exceptional results.
Key Benefits of Custom Training
Training ChatGPT or LLMs on your company's data offers several compelling advantages:
1. Improved Accuracy
By using your data, you fine-tune the models to understand your industry-specific terms, jargon, and context. They become adept at handling complex queries, providing insightful answers, and setting you apart as a market leader.
2. Higher Customer Retention
Custom training for customer interactions, personalized offerings, and customer service creates unique experiences, keeping your customers coming back.
Customers have become more sensitive to their user journey. You could retain up to 78% of consumers if they had an amazing customer experience on a digital channel, while 64% will switch to a competitor if they suffer from a poor customer experience.
3. Enhanced Personalization
Inject your brand's tone and values into the models for content that aligns perfectly with your brand identity, ensuring a personalized experience.
4. Increased Efficiency
Models trained on your data streamline processes, automating repetitive tasks, saving time, and freeing up employees for more valuable activities. It enhances productivity and innovation.
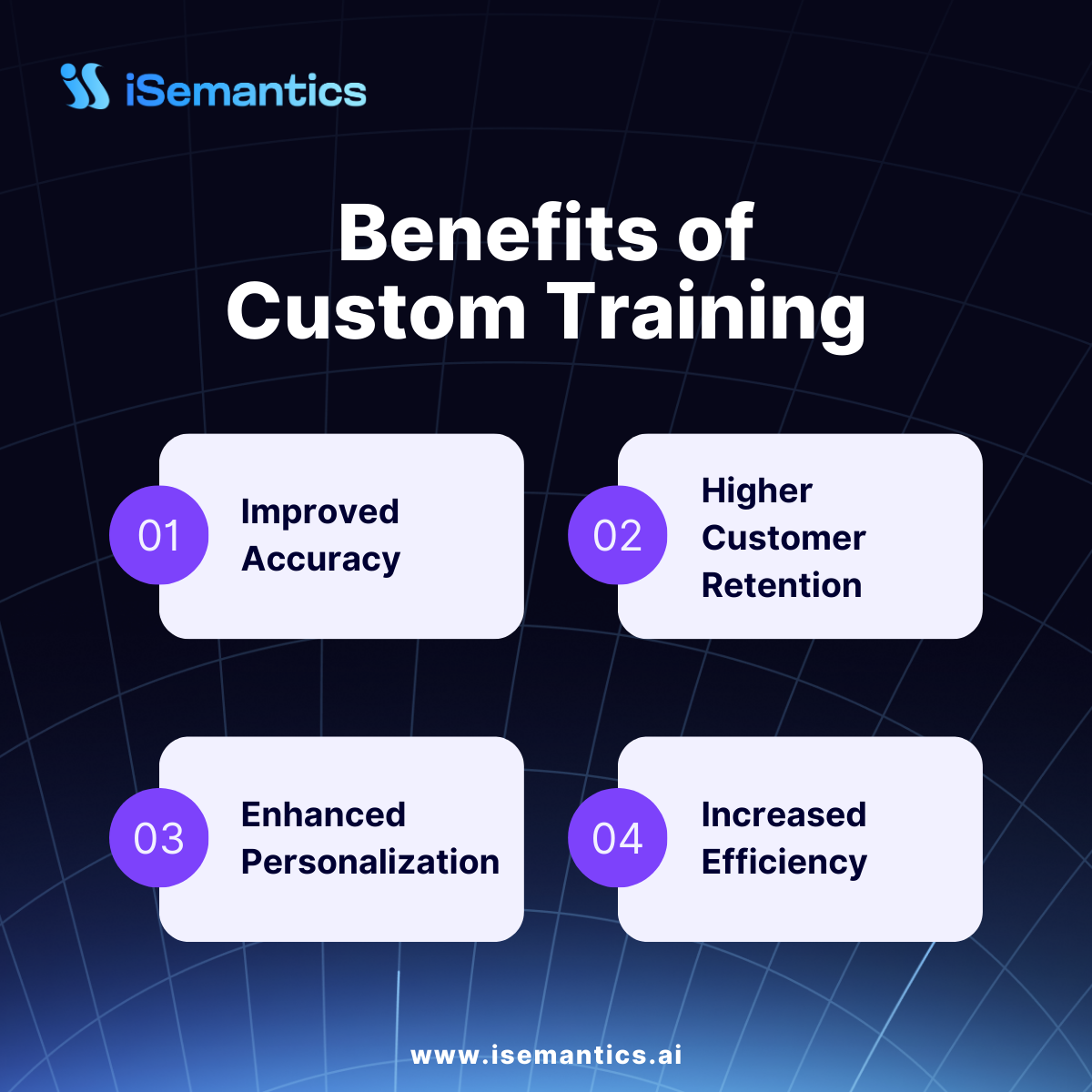
How to Train ChatGPT or LLMs on Custom Data
Training ChatGPT or LLMs on your business data may seem like a daunting task, but it can be accomplished with the right approach. Here's a step-by-step plan:
1. Data Collection
Collect diverse data from within your organization, including customer support tickets, product documentation, and internal knowledge bases. The more diverse and comprehensive your data, the better.
Additionally, don't limit yourself to just recent data. It is valuable to include historical data as well, as it contains insights into long-term trends and patterns.
2. Data Preparation
Clean and organize your data to remove any sensitive or irrelevant information. This step is crucial to ensure the models' accuracy and reliability.
During the data preparation stage, it's important to filter out irrelevant data such as spam, duplicate entries, or data that is not representative of the task you want to train the model for. Ensure that you also remove sensitive information to ensure compliance with data protection regulations.
Keep in mind that training LLMs is a transformation process. The quality of the output depends highly on the quality of the input. The cleaner and better labeled your input data is, the more relevant and accurate the output will be.
3. Model Training
Once the training data is set, it is time to choose the most adequate LLM that will allow you to achieve the highest accuracy quickly and with minimal cost. Once selected, you’ll need to feed the model with your business data and fine-tune its parameters to optimize performance.
When training the model, you should consider the computational resources required. Depending on the size of your dataset and the complexity of the models, you will need to allocate sufficient computing power to ensure efficient training.
Next, experiment with different hyperparameters and training strategies to find the optimal configuration for your specific use case. This will involve using a combination of LLMs and adjusting learning rates, batch sizes, and the number of training iterations to enhance performance and achieve better results.
4. Evaluation and Iteration
Continuous evaluation and refinement of the trained models are essential to consistently enhance accuracy, enabling them to continually learn and understand the patterns.
Once the models are trained, evaluate their performance on a separate validation dataset. This dataset should contain examples that were not used during the training process so that you assess the models' generalization capabilities.
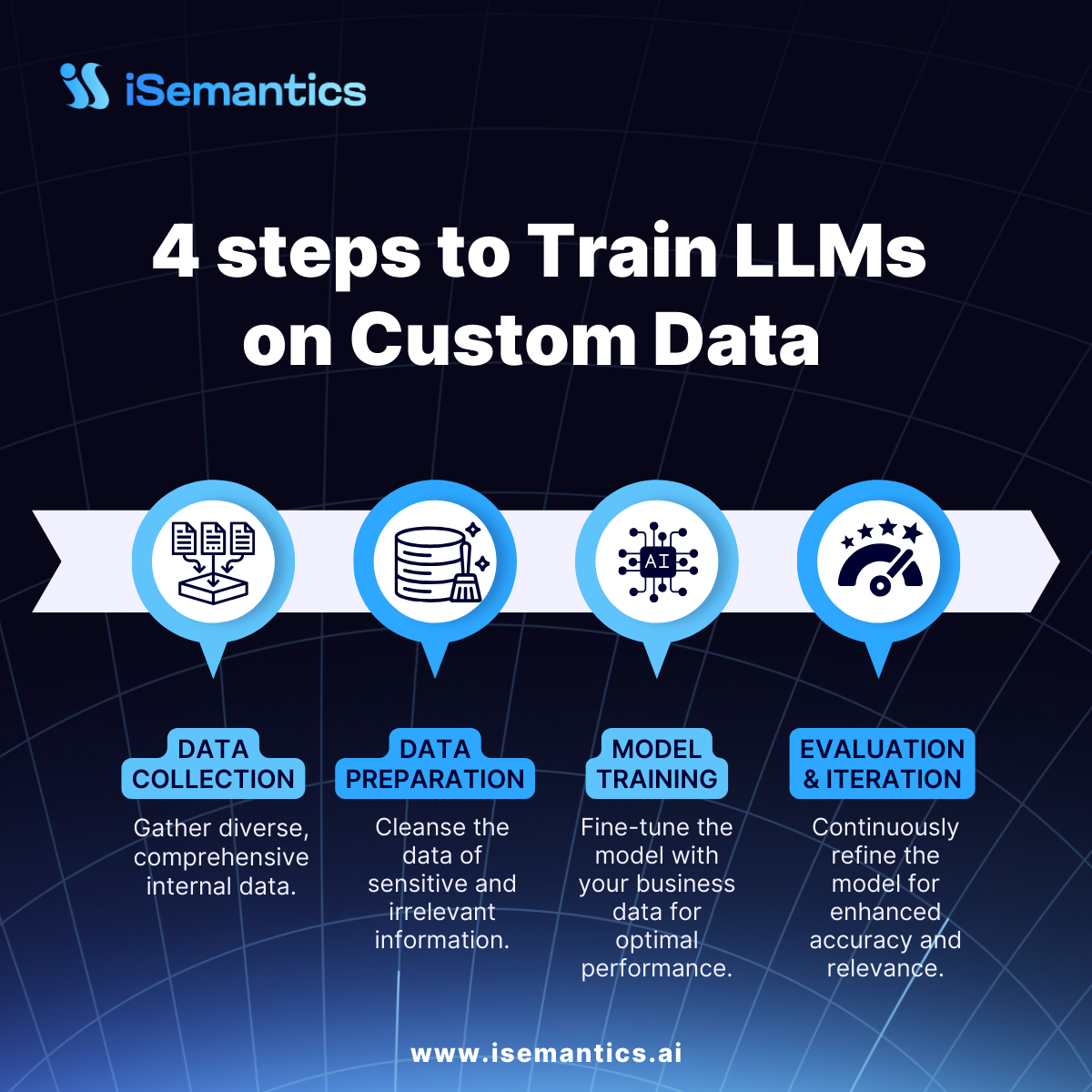
The Limits of LLMs
While training ChatGPT or LLMs on your business data brings numerous benefits, it is essential to acknowledge their limits:
1. Data Privacy
When you prepare datasets to train LLMs you must be careful not to share proprietary information or any personally identifiable information (PII) that could violate privacy regulations like GDPR or CCPA.
2. Domain-Specific Focus
LLMs trained on your company's data may not be proficient in answering queries outside of your specific domain. For broader topics, additional training or utilizing LLMs with wider pre-training will be necessary.
3. Output Quality
The quality and relevance of your company's data are pivotal for effective training. Ensure that the data used in training is structured, accurate, and aligned with your intended results.
Unstructured or biased data will automatically impede your model performance and lead to “hallucinations” and inaccurate responses. Such errors will be problematic to your business.
4. Ongoing Updates
ChatGPT and any other LLMs require continuous monitoring and updates to adapt to changing trends, customer preferences, and industry dynamics. Regular fine-tuning and optimization are necessary to maintain peak performance.
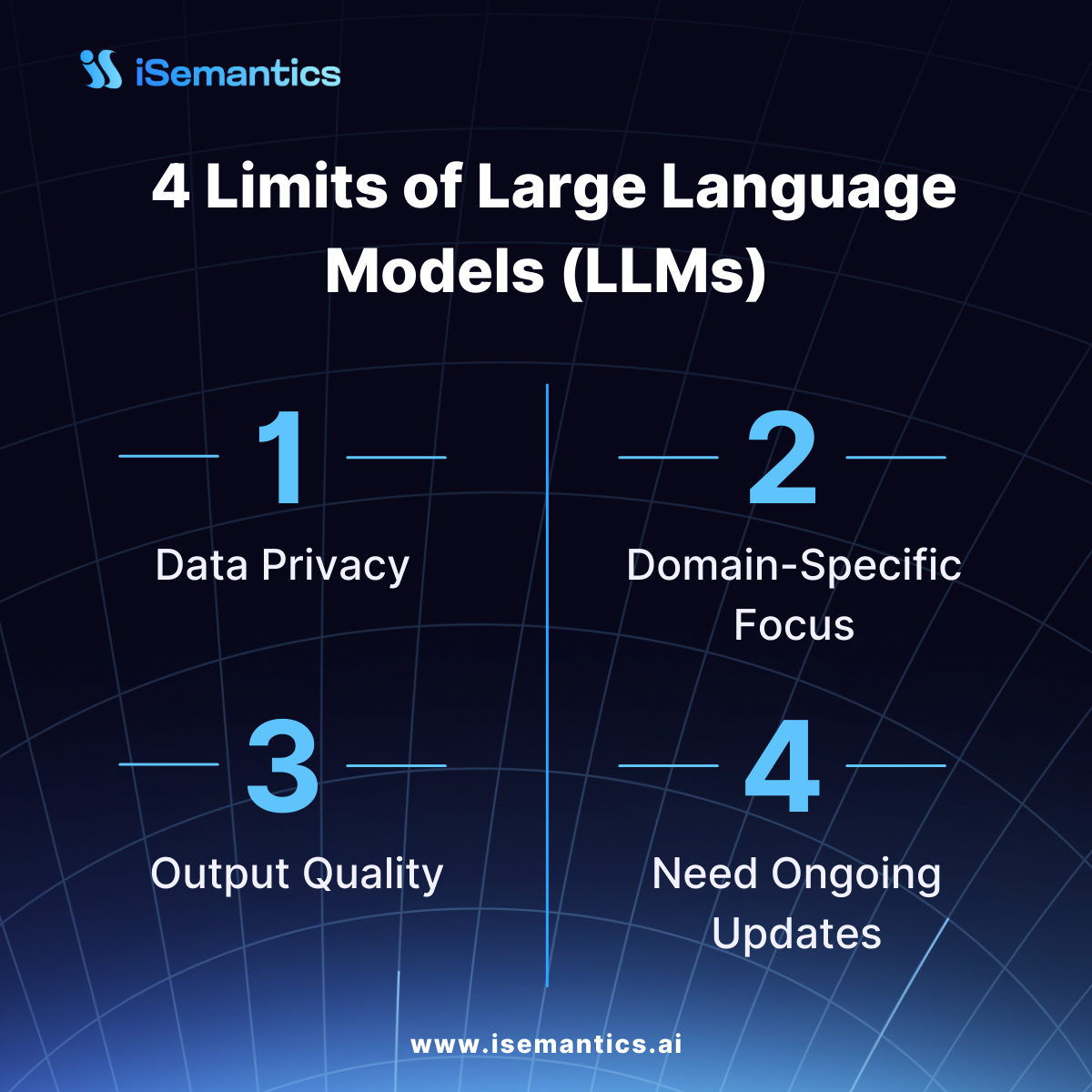
Final Thoughts
LLMs, especially ChatGPT, are transforming industries across the board. Now, the power is in your hands.
If you're considering integrating LLMs into your business operations, we recommend you start with a proof-of-concept (POC) using non-sensitive data. The data points needed are only a few hundred instead of millions, and the results are ready in a matter of days instead of months. It's a quick way to test the waters, assess the AI project’s potential, estimate the costs, and address any data privacy or security concerns.
Your journey towards a brighter AI-driven future begins with iSemantics. Schedule a free consultation call with our team to build a quick affordable POC and get ahead of everybody else.